In the realm of Artificial Intelligence (AI), the spotlight often gravitates towards Large Language Models (LLMs), chatbots, and Generative AI. However, the scope of AI extends far beyond these applications, encompassing invaluable techniques like machine learning, deep learning, and predictive modeling. This article, authored by Daniele Micci, a seasoned expert in Data Science and an integral part of Neodata Group’s Advisors Board, delves into the multifaceted landscape of AI and its diverse applications in business.
Artificial intelligence (AI) has become a very popular topic in recent years, with many people associating it with Large Language Models (LLMs), chatbots, and Generative AI. However, there is much more to AI than just these specific applications. Machine learning, deep learning, and other types of predictive modeling methods are extremely valuable in many business applications.
Machine learning (ML) is a type of AI that allows computers to learn without being explicitly programmed. ML models are trained on data, and they learn to identify patterns and relationships in that data. Once trained, ML models can be used to make predictions about new data.
Deep learning is a type of ML that uses artificial neural networks to learn from data. Neural networks are inspired by the human brain, and they are able to learn complex patterns and relationships in data. Deep learning models are often used and extremely effective for tasks such as image recognition, natural language processing, and speech recognition.
Predictive modeling tools are used to make predictions about future events or outcomes. Predictive modeling tools can use a variety of techniques, including machine learning and deep learning. Predictive modeling tools are used in many different business applications, such as customer churn prediction, fraud detection, and demand forecasting.
Examples of Machine Learning models in business:
- Customer churn prediction: ML models can be used to predict which customers are likely to churn (cancel their subscription or service). This information can be used to develop targeted retention campaigns.
- Fraud detection: ML models can be used to detect fraudulent transactions and activities. This information can be used to protect businesses from financial losses.
- Demand forecasting: ML models can be used to forecast demand for products and services. This information can be used to make better inventory and production decisions.
- Product recommendation: ML models can be used to recommend products to customers based on their past purchase history and other factors. This can help businesses to increase sales and improve the customer experience.
- Pricing: ML models can be used to set optimal prices for products and services. This can help businesses to maximize profits and increase sales.
LLMs represent the latest AI technology and are essentially extremely large ML models trained on enormous collections of textual content – these models can process textual inputs and build an effective internal representation of the “knowledge” contained in the textual content they are trained on. Aside from enabling conversational applications, such as chatbots, LLMs can be used in combination with traditional ML models in their most common business applications. For example:
- LLMs can be used to generate features, or inputs, for traditional ML models. For example, an LLM could be used to generate a summary of a customer review, and this summary could then be used as input to a machine-learning model that predicts customer churn.
- LLMs can be used to create or optimize new machine-learning models. For example, an LLM could be used to generate a new type of neural network architecture that is specifically designed for a particular task, such as customer segmentation or product recommendation.
Overall, AI is a powerful tool that can be used to improve many different business processes. Machine learning, deep learning, and other types of predictive modeling tools are already being used by businesses of all sizes. LLMs are a newer type of AI, but they have the potential to be even more powerful when used in combination with traditional machine learning models.
AI is a rapidly developing field, and new applications are being discovered all the time. Businesses that are able to effectively implement AI will have a significant advantage over their competitors.
To explore how AI can elevate your business processes connect with Neodata Group. For more information and tailored insights, reach out at info@neodatagroup.ai. Unlock the potential of AI and propel your business into the future.
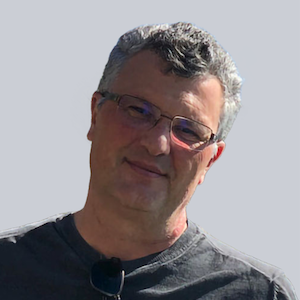
Daniele Micci Barreca
Daniele Micci Barreca is a Senior Staff Data Scientist at Google, currently leading a high-performing product analytics team within the Google Search organization.
Prior to his role at Google, Daniele led a payments analytics team at Uber, focusing on optimization and reliability efforts that significantly enhanced Uber's payments infrastructure. Daniele co-founded Elite Analytics, a data science consulting firm. In this role, he played a pivotal part in developing machine learning systems for tax compliance applications.
Daniele's academic journey includes a Ph.D. in Cognitive and Neural Systems from Boston University, specializing in cognitive science, and a Master's degree in Computer Science from the University of Houston.
-
This author does not have any more posts.